Note
Go to the end to download the full example code.
VES inversion for a smooth model#
This tutorial shows how an built-in forward operator is used for an Occam type (smoothness-constrained) inversion with fixed regularization (most natural). A direct current (DC) one-dimensional (1D) VES (vertical electric sounding) modelling operator is used to generate data, add noise and inversion.
We import numpy numerics, mpl plotting, pygimli and the 1D plotting function
import numpy as np
import matplotlib.pyplot as plt
import pygimli as pg
from pygimli.physics.ves import VESRhoModelling, VESManager
from pygimli.viewer.mpl import drawModel1D
Set up synthetic model and simulate data
synRes = [100., 500., 20., 800.] # synthetic resistivity
synThk = [4, 6, 10] # synthetic thickness (lay layer is infinite)
ab2 = np.logspace(-1, 2, 25) # 0.1 to 100 in 25 steps (8 points per decade)
ves = VESManager()
rhoa, error = ves.simulate(synThk+synRes, ab2=ab2, mn2=ab2/3,
noiseLevel=0.03, seed=1337)
Set up the forward operator
Set up inversion
inv = pg.Inversion(fop=f, verbose=False)
# inv.setRegularization(cType=1) # default = not necessary
Create some transformations used for inversion
inv.transData = pg.trans.TransLog() # log transformation also for data
inv.transModel = pg.trans.TransLogLU(1, 1000) # lower and upper bound
Set pretty large regularization strength and run inversion
print("inversion with lam=100")
res100 = inv.run(rhoa, error, lam=100)
print('rrms={:.2f}%, chi^2={:.3f}'.format(inv.relrms(), inv.chi2()))
inversion with lam=100
rrms=5.15%, chi^2=2.951
Decrease the regularization (smoothness) and repeat
print("inversion with lam=10")
res10 = inv.run(rhoa, error, lam=10)
print('rrms={:.2f}%, chi^2={:.3f}'.format(inv.relrms(), inv.chi2()))
inversion with lam=10
rrms=3.43%, chi^2=1.309
Decrease the regularization (smoothness) and repeat
print("inversion with second order smoothness")
inv.setRegularization(cType=2)
resC2 = inv.run(rhoa, error, lam=20)
print('rrms={:.2f}%, chi^2={:.3f}'.format(inv.relrms(), inv.chi2()))
inversion with second order smoothness
rrms=3.06%, chi^2=1.038
Show model (inverted and synthetic) as well as model response and data
fig, ax = plt.subplots(ncols=2, figsize=(8, 6)) # two-column figure
drawModel1D(ax[0], synThk, synRes, color='C0', label='synthetic',
plot='semilogx')
drawModel1D(ax[0], thk, res100, color='C1', label=r'$\lambda$=100')
drawModel1D(ax[0], thk, res10, color='C2', label=r'$\lambda$=10')
drawModel1D(ax[0], thk, resC2, color='C4', label=r'C=2')
ax[0].grid(True, which='both')
ax[0].legend(loc='best')
ax[1].loglog(rhoa, ab2, 'x-', color="C0", label='measured')
ax[1].loglog(inv.response, ab2, '-', color="C5", label='fitted')
ax[1].set_ylim((max(ab2), min(ab2)))
ax[1].grid(True, which='both')
ax[1].set_xlabel(r'$\rho_a$ [$\Omega$m]')
ax[1].set_ylabel('AB/2 [m]')
ax[1].yaxis.set_label_position('right')
ax[1].yaxis.set_ticks_position('right')
ax[1].legend(loc='best');
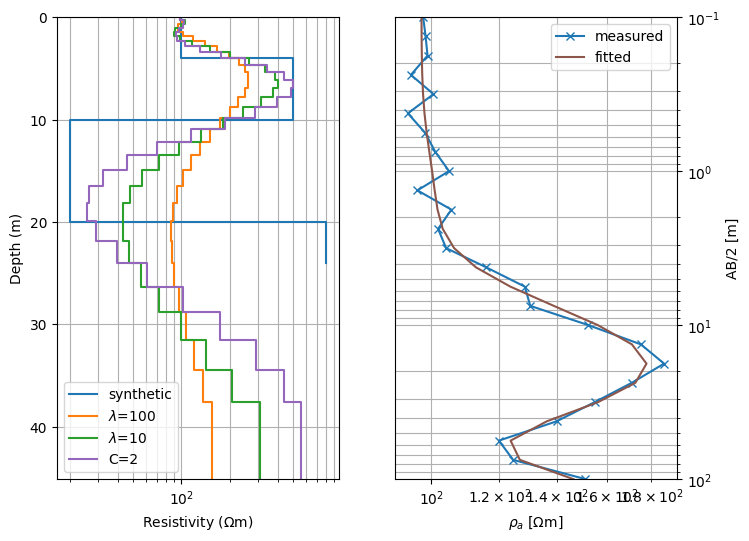
<matplotlib.legend.Legend object at 0x7f978e0a3050>